Latest from 5G/6G & Fixed Wireless Access/Mobile Evolution
What You Need to Know About Nvidia's 6G Research Platform
With 5G rollouts still fresh in our memory, the industry is already working towards the next generation, or 6G. There’s a lot of groundwork to be done before we’re living in a 6G world, though.
Nvidia, the GPU company that’s become probably the biggest name in AI hardware, knows this, and is already making a name for itself in 6G, knowing that AI is likely to be far more integral to the RAN than we might have predicted ten years ago.
Nvidia’s 6G Research Cloud Platform is a platform that includes an “Aerial Omniverse Digital Twin” for simulating 6G environments in the real world and in real time so developers and engineers can test and optimize networks (using the power of AI, of course), and other features available to those working on the genesis era of the new technology.
“We want to be the canvas for the da Vincis of the world."
Nvidia’s platform is intended for the very early stages, or pre-standardization of 6G. In other words, the engineers, developers, and academics who are in the early stage of figuring out how to make 6G work.
“We want to be the canvas for the da Vincis of the world,” says Soma Velayutham, AI & Telco Lead at Nvidia. Velayutham told ISE Magazine that they’ve been getting hundreds of requests for the platform.
Nvidia and the AI Knowledge Gap
One problem that almost every industry is facing, is that engineers and developers who may have deep knowledge of a vast number of technologies and tools, may not have the AI expertise needed to jump into it, since the technology behind the latest models is so new.
“Most of the radio engineers don’t understand AI algorithms,” he says. “They can write some code, but they don’t know how to optimize it, they don’t understand how to make it super-efficient and low energy, low latency … so we have tools that are designed to optimize codes that researchers create.”
And 6G is going to require very specific and advanced uses of AI. “Because wireless is almost real time, you can’t tolerate more than 30 milliseconds of delay, any inferencing architecture, any AI algorithm you put in, has to be super low latency. The model size matters, the energy efficiency matters…”
The Nvidia platform is trying to solve this, as well as offer a framework that can be used however it might be needed.
“We want to make sure that we have all the tools for an AI/ML engineer who may not really understand radio. And bridge the gap between the radio engineering world and the AI/ML world,” he says.
To neatly sum up the platform and its purpose, Velayutham refers to the three “pillars” it’s built upon.
“The 6G research cloud platform has three pillars to it. The first is a software-defined stack the researchers can take advantage of. We provide full source code to anybody who wants. They can take it, they can rip it apart, they can replace it, they can do all kinds of things. The second part of it is a simulation environment, and the third is the AI frameworks that we provide.”
Simulating 6G in Real-World Environments
To deal with the complexities and variables of real-world environments, such as a big city, that’s where the Aerial Omniverse Digital Twin comes in.
Building radio networks in cities comes with obvious challenges. And to add to that, the industry believes that smart cities are the next phase of urban evolution, and more and more devices, including vehicles, will be connected to the internet. Planning for these unknowns is difficult, but necessary.
Velayutham notes that current simulations are really only site specific, but the Nvidia platform goes beyond this to address very large urban areas.
“We can actually do a city-scale simulation. So you can take the entire [city of] New York, and actually deploy one million cell phones and users and see what happens to the network.” The idea is to simulate as much detail as possible. “Omniverse is physics compliant,” he adds. “It’s real time, so you can have material properties, so concrete behaves like concrete, glass behaves like glass.”
The project’s wireless team created an electromagnetic propagation layer to stack on existing layers. “If you had a beam in New York, and you had concrete and you had snow, how does it behave? And you can change that and say ‘what happens in summer? What happens if I reduce the antennae height?’”
These real-world, wireless environments will require cutting-edge data transfer capabilities, of course. In these network environments, they “can’t tolerate more than 30 milliseconds of delay. Any inferencing architecture, any AI algorithm you put in, has to be super low latency, the model size matters, the energy efficiency matters…”
Importing Data
To get the details required to simulate a site or area, an engineer can import various maps and other information into the platform. “If you go into a city, you can take the lidar and open-source map, import it into Omnibus. You can take the AutoCAD drawing of your building and import that into the Omniverse. So you can actually have a real simulation of a real environment by importing all these assets.”
The Future of RAN Engineering
Velayutham talks about the role of AI in planning the network, using the language of federal, county, and local.
“The best way to describe it is you have the city, the county, and the federal. The city has to optimize itself and do the work itself, it has to be efficient in itself. Then you have the county, multiple cities that have to come together, then you have the state and federal. So it’s a little bit of a hierarchy of optimization. AI helps in all layers.”
And when you zoom in, the RAN challenges become complicated and specific, because you’re dealing with something unique at each location.
“The biggest opportunity is actually the local level. Because that’s where the spectrum is being utilized. The higher you go up, the more general the optimization, the easier it is to do, but the less benefit you get. The more you go to the local, it’s harder to do because it’s very site specific, but that’s where you get the largest gain.”
Velayutham is confident that Nvidia’s platform will enable this future of 6G combined with AI. Right now, it’s meant to be used as a starting point for researchers, developers, and academics. It’s something Nvidia sees other organizations using to start building their own commercial platforms on.
Nvidia sees the platform as an investment that will help the industry in the years to come, with the proliferation of connected devices, the growth of AI tools, and general consumer and business expectations and the bandwidth demand that’s set to keep growing.
“Most of the people we talk to are delighted that we are investing to create tools and creating an infrastructure for researchers to do their work.”
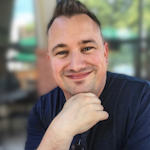
Joe Gillard | Executive Editor
Joe Gillard is a media professional with over 10 years of experience writing, editing, and managing the editorial process across a spectrum of innovative industries. Joe strives to deliver the best possible editorial product by focusing on the needs of the audience, utilizing the data available, and collaborating with a talented team.