Latest from Network Transformation/Edge Compute/IoT/URLLC/Automation/M2M
You’re Ready for AI, But is Your Data?
Establishing a strong foundation for AI starts with high-quality network data.
Fiber operators face increasing pressure to adopt emerging technologies to stay competitive as the industry evolves. A recent report by McKinsey & Company emphasizes the positive impact that AI can have on the telecommunications industry in terms of growth. As put in the report, “evidence suggests that AI could be the catalyst to reignite growth after a decade of stagnation.”
AI offers significant benefits, such as self-healing infrastructure and touchless customer service. It can transform predictive maintenance by analyzing network data to foresee equipment failures, thus reducing costly downtime and optimizing resource allocation. Additionally, AI-driven algorithms can greatly enhance the performance, efficiency and reliability of fiber networks by identifying bottlenecks, suggesting capacity upgrades, and improving service quality. However, the successful deployment of AI hinges on the quality of the underlying fiber network data.
To fully leverage AI and ML, fiber operators should begin by prioritizing strategies and technologies that create a comprehensive and accurate network model.
The Importance of Network Data Quality
For broadband operators, having a robust and accurate representation of their physical network infrastructure is vital for business success and is a critical foundation to leverage AI technology. High-quality data on asset locations, connections, and configurations is essential for operational efficiency, informed decision-making, and cost optimization.
Unfortunately, fiber network operators often encounter significant data quality challenges, such as inaccurate or incomplete asset data, inconsistent network documentation, and data silos. These issues can lead to errors in network planning, increased construction and maintenance costs, and difficulties in integrating emerging technologies like AI. The dynamic nature of fiber networks further complicates maintaining data accuracy.
To fully leverage AI and ML, fiber operators should begin by prioritizing strategies and technologies that create a comprehensive and accurate network model. This includes:
- Holistic Asset Management: Establishing a centralized repository for detailed asset information, including location, type, and connectivity.
- Dynamic Network Documentation: Implementing field processes for continuous data updates to reflect network changes and expansions.
- Data Integration: Breaking down data silos to create a unified view of the network for improved analysis and decision-making.
Optimizing the Network
A physical network is at the core of any telecom organization. Operators should efficiently plan, design, build, operate, and monetize their networks to accelerate ROI. Initially, this may involve replacing spreadsheets and CAD drawings with fiber network documentation software. However, many larger, established broadband operators still use outdated geographic information systems and a collection of siloed applications. This may result in more agile operators with integrated life cycle management solutions optimized for fiber networks outpacing these larger incumbents.
Prioritizing an optimized fiber network model, such as a digital twin built specifically for the network, prevents the creation of a complicated and fragmented software infrastructure that is difficult to support, upgrade, and evolve. An integrated network management strategy enables operators to lay a strong foundation, so when they decide to invest in emerging AI and ML technologies, they are well-positioned to take advantage of the business optimization benefits offered by advanced technology.
In Preparation for Emerging Technologies
To prioritize data quality and prepare for AI and ML, telecom operators should consider the following steps:
- Assess Data Health: Examine the accuracy and completeness of network data to pinpoint any issues impacting operations.
- Integrate Data Management: Combine separate data systems into a single, integrated platform for efficient network management.
- Establish Data Standards: Create clear rules for data handling to ensure accuracy, consistency, and speedy updates.
- Partner for Success: Collaborate with technology experts to optimize network data collection and management.
- Continuously Improve Data Quality: Regularly review and enhance data quality through ongoing updates and process refinements.
Picking the Right Partners for the Journey
Working with a primary technology vendor who understands the operator's fiber network model and life cycle requirements often proves more efficient than working with multiple vendors. Establishing robust relationships with a select few vendors creates a strong foundation for the long-term management of the fiber network.
This strategy facilitates seamless integration of essential software components and ensures strategic alignment among all stakeholders involved, simplifying management, delivering significant ROI, and setting the business up for future success.
As the telecommunications industry evolves, the landscape of network data management will also transform. Advanced data analytics, sophisticated data governance frameworks, and robust data privacy measures will play crucial roles in shaping future network operations.
AI and ML will be central to these advancements, driving predictive analytics, automated data quality improvements, and optimized network performance. By embracing these technologies and best practices, telecom operators can extract greater value from their network data, streamline operations, and secure a competitive advantage in the rapidly changing telecommunications market.
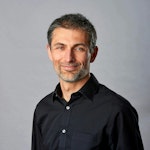
David Cottingham | CTO, IQGeo
David Cottingham is CTO at IQGeo, and is an experienced product leader in enterprise SaaS, API integrations, and cloud infrastructure. He holds a Ph.D in Computer Science from the University of Cambridge. For more information, visit www.iqgeo.com. Follow David on LinkedIn. Follow IQGeo on LinkedIn and X @IQGeo_software.